Note
Go to the end to download the full example code
Univariate Gaussian Quadrature
Univariate Gaussian quadrature can be used to efficiently integrate smooth one-dimensional functions. While numpy supports Hermite and Legendre Gaussian qurature, Pyapprox can generate Gaussian quadrature rules for any continouous random variable implemented in scipy.stats.
To generate a univariate quadrature rule for uniform random variables
from pyapprox import surrogates
from scipy import stats
degree = 10
scipy_var = stats.uniform(-1, 2)
quad_rule = surrogates.get_gauss_quadrature_rule_from_marginal(
scipy_var, degree+1)
x_quad, w_quad = quad_rule(5)
As an example, we can use this quadrature rule to integrate \(\rv^2\) with repsect to the uniform PDF on [-1, 1], i.e. 1/2
values = x_quad**2
integral = values.dot(w_quad)
print(integral)
0.3333333333333332
The quadrature recovers the integral value of 1/3 to machine precision. Note
unlike leggauss
we are integrating with
resepect to the uniform PDF.
The function is also capable of generating rules on different intervals For example
scipy_var = stats.uniform(0, 2)
x_quad, w_quad = surrogates.get_gauss_quadrature_rule_from_marginal(
scipy_var, degree+1)(degree)
values = x_quad**2
integral = values.dot(w_quad)
print(integral)
1.3333333333333346
Quadrature rules can be created for almost any random variable. Here we will generate a quadrature rule for an exponential random variable
scipy_var = stats.expon()
x_quad, w_quad = surrogates.get_gauss_quadrature_rule_from_marginal(
scipy_var, degree+1)(degree)
values = x_quad**2
integral = values.dot(w_quad)
print(integral)
2.000000000000001
For interest, we plot the quadrature rule against the PDF of the exponential variable
import numpy as np
import matplotlib.pyplot as plt
from pyapprox.analysis import visualize
from pyapprox.variables import marginals
visualize.plot_discrete_measure_1d(x_quad, w_quad)
vrange = marginals.get_truncated_range(scipy_var, 1-1e-6)
xx = np.linspace(vrange[0], vrange[1], 101)
plt.fill_between(xx, 0*xx, scipy_var.pdf(xx), alpha=0.3)
plt.show()
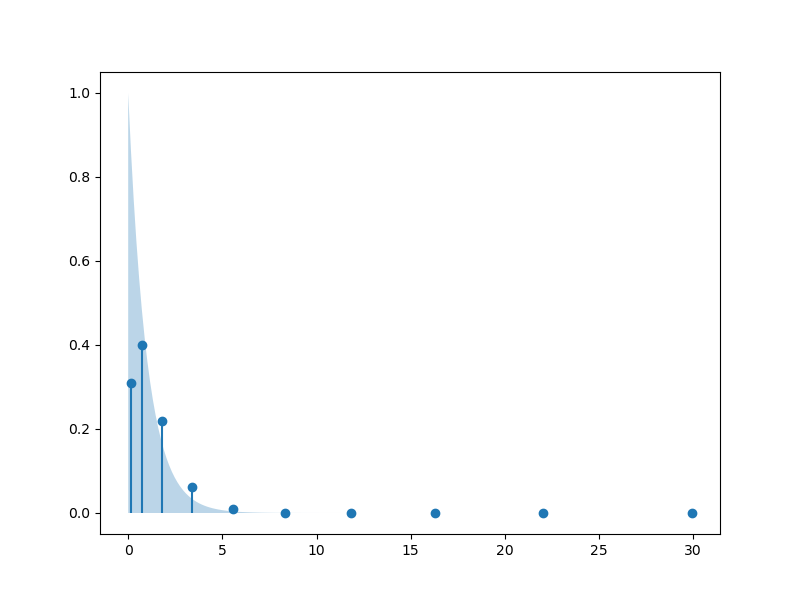
Total running time of the script: ( 0 minutes 0.098 seconds)