[1]:
%matplotlib inline
%load_ext autoreload
%autoreload 2
[2]:
import numpy as np
import matplotlib.pyplot as plt
from helpr.physics.api import CrackEvolutionAnalysis
from helpr.utilities.unit_conversion import convert_psi_to_mpa, convert_in_to_m
from probabilistic.capabilities.uncertainty_definitions import UniformDistribution, NormalDistribution, DeterministicCharacterization
[3]:
# # turn warnings back on for general use
# import warnings
# warnings.filterwarnings('ignore')
Inspection Mitigation Analysis of Pipeline Population
Problem Specification
Geometry
[4]:
pipe_outer_diameter = DeterministicCharacterization(name='outer_diameter',
value=convert_in_to_m(36)) # 36 inch outer diameter, m
wall_thickness = DeterministicCharacterization(name='wall_thickness',
value=convert_in_to_m(0.406)) # 0.406 inch wall thickness, m
Material Properties
[5]:
yield_strength = DeterministicCharacterization(name='yield_strength',
value=convert_psi_to_mpa(52_000)) # material yield strength of 52_000 psi, MPa
fracture_resistance = DeterministicCharacterization(name='fracture_resistance',
value=55) # fracture resistance (toughness), MPa m1/2
Operating Conditions
! 85 ksi pressure cause hoop stresses to exceed allowable stresses
[6]:
max_pressure = NormalDistribution(name='max_pressure',
uncertainty_type='aleatory',
nominal_value=convert_psi_to_mpa(840),
mean=convert_psi_to_mpa(850),
std_deviation=convert_psi_to_mpa(20)) # maximum pressure during oscillation, MPa
min_pressure = NormalDistribution(name='min_pressure',
uncertainty_type='aleatory',
nominal_value=convert_psi_to_mpa(638),
mean=convert_psi_to_mpa(638),
std_deviation=convert_psi_to_mpa(20)) # minimum pressure during oscillation, MPa
temperature = UniformDistribution(name='temperature',
uncertainty_type='aleatory',
nominal_value=293,
upper_bound=300,
lower_bound=285) # gas blend temperature variation, K
volume_fraction_h2 = UniformDistribution(name='volume_fraction_h2',
uncertainty_type='aleatory',
nominal_value=0.1,
upper_bound=0.2,
lower_bound=0) # % volume fraction H2 in natural gas blend, fraction
Initial Crack Dimensions
[7]:
flaw_depth = UniformDistribution(name='flaw_depth',
uncertainty_type='aleatory',
nominal_value=25,
upper_bound=30,
lower_bound=20) # population of flaw % through pipe thickness, %
flaw_length = DeterministicCharacterization(name='flaw_length',
value=convert_in_to_m(1.575)) # length of initial crack/flaw, m
Quantities of Interest (QoI)
[8]:
plotted_variable = 'Cycles to a(crit)'
Probabilistic Settings
[9]:
sample_type = 'lhs'
sample_size = 10_000
Analysis
Using LHS sampling of uncertain variables
[10]:
analysis = CrackEvolutionAnalysis(outer_diameter=pipe_outer_diameter,
wall_thickness=wall_thickness,
flaw_depth=flaw_depth,
max_pressure=max_pressure,
min_pressure=min_pressure,
temperature=temperature,
volume_fraction_h2=volume_fraction_h2,
yield_strength=yield_strength,
fracture_resistance=fracture_resistance,
flaw_length=flaw_length,
aleatory_samples=sample_size,
sample_type=sample_type)
analysis.perform_study()
/Users/bbschro/Development/helpr/src/helpr/physics/stress_state.py:348: UserWarning: Inner Radius / wall thickness exceeds bounds 5 <= R_i/t <= 20, max R_i/t = 43.33497536945812, violating Anderson solution limits.
wr.warn('Inner Radius / wall thickness exceeds bounds ' +
/Users/bbschro/Development/helpr/src/helpr/physics/stress_state.py:129: UserWarning: Stress state exceeding 72% SMYS
wr.warn('Stress state exceeding 72% SMYS', UserWarning)
/Users/bbschro/Development/helpr/src/helpr/physics/stress_state.py:348: UserWarning: Inner Radius / wall thickness exceeds bounds 5 <= R_i/t <= 20, max R_i/t = 43.33497536945812, violating Anderson solution limits.
wr.warn('Inner Radius / wall thickness exceeds bounds ' +
Inspection Mitigation
[11]:
probability_of_detection = 0.8 # 80%
detection_resolution = 0.3 # able to detect cracks greater than 30% through
inspection_interval = 4 # how many years between inspections
inspection_frequency = 365*inspection_interval # inspections in terms of cycles
criteria='Cycles to a(crit)'
mitigated = analysis.apply_inspection_mitigation(probability_of_detection,
detection_resolution,
inspection_frequency,
criteria)
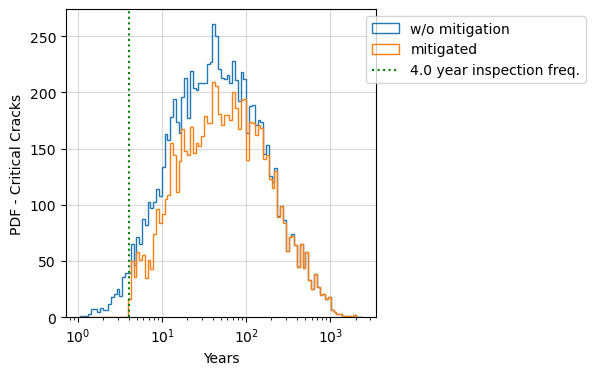
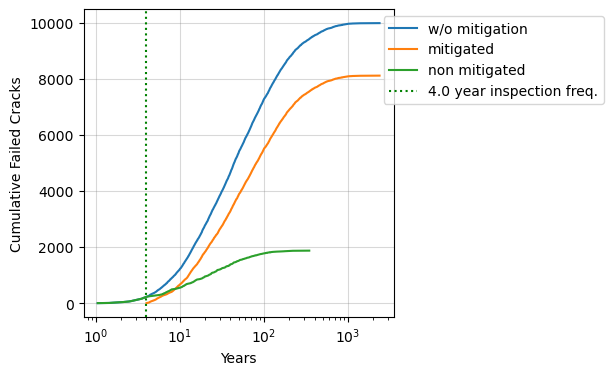
[12]:
percent_mitigated = sum(mitigated)/sample_size*100
print(f'Percent mitigated through inspection = {percent_mitigated:.1f}%')
Percent mitigated through inspection = 81.5%
[13]:
def plot_log_hist(data, label, logbins=None):
if logbins is None:
num_bins = int(np.sqrt(data.size))
hist, bins = np.histogram(data, bins=num_bins)
logbins = np.logspace(np.log10(bins[0]), np.log10(bins[-1]), len(bins))
plt.hist(x=data,
bins=logbins,
histtype='step',
density=False,
label=label)
return logbins
[14]:
cycle_life_data = analysis.life_criteria[criteria][0]/365
nominal_life_result = analysis.nominal_life_criteria[criteria][0]/365
mitigated_life_data = cycle_life_data[mitigated]
not_mitigated_life_data = cycle_life_data[np.invert(mitigated)]
plt.figure(figsize=(4, 4))
logbins = plot_log_hist(cycle_life_data, 'raw data')
plot_log_hist(mitigated_life_data, 'mitigated', logbins)
ylims = plt.gca().get_ylim()
plt.plot([nominal_life_result]*2,
ylims,
'r--',
label='nominal')
plt.plot([inspection_interval]*2,
ylims,
'g:',
label=f'{inspection_interval} year inspection freq.')
legend = plt.legend(loc='upper right', bbox_to_anchor=(1.5, 1))
plt.xlabel('Years')
plt.ylabel('PDF')
plt.xscale('log')
plt.grid(color='gray', alpha=0.3)
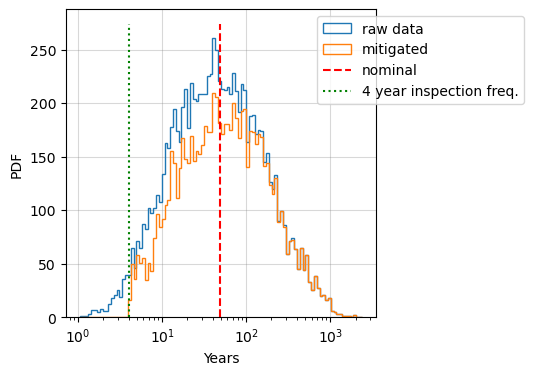
[15]:
plt.figure(figsize=(4, 4))
plt.plot(np.sort(cycle_life_data),
np.arange(len(cycle_life_data))/len(cycle_life_data), label='raw data')
plt.plot(np.sort(mitigated_life_data),
np.arange(len(mitigated_life_data))/len(mitigated_life_data), label='mitigated')
plt.plot(np.sort(not_mitigated_life_data),
np.arange(len(not_mitigated_life_data))/len(not_mitigated_life_data), label='non mitigated')
plt.plot([inspection_interval]*2,
[0, 1],
'g:',
label=f'{inspection_interval} year inspection freq.')
legend = plt.legend(loc='upper right', bbox_to_anchor=(1.5, 0.5))
plt.xlabel('Years')
plt.ylabel('CDF')
plt.xscale('log')
plt.grid(color='gray', alpha=0.3)
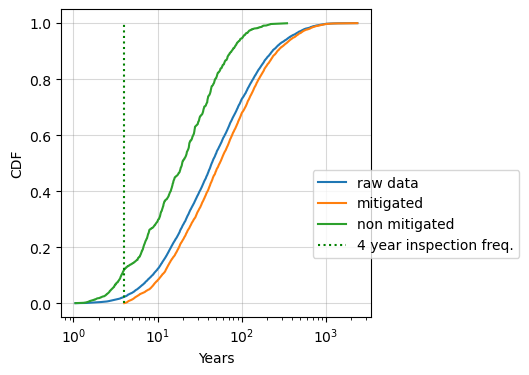
[16]:
plt.figure(figsize=(4, 4))
plt.plot(np.sort(cycle_life_data),
np.arange(len(cycle_life_data)), label='raw data')
plt.plot(np.sort(mitigated_life_data),
np.arange(len(mitigated_life_data)), label='mitigated')
plt.plot(np.sort(not_mitigated_life_data),
np.arange(len(not_mitigated_life_data)), label='non mitigated')
legend = plt.legend(loc='upper right', bbox_to_anchor=(1.5, 0.5))
plt.xlabel('Years')
plt.ylabel('Cumulative Failed Cracks')
plt.xscale('log')
plt.grid(color='gray', alpha=0.3)
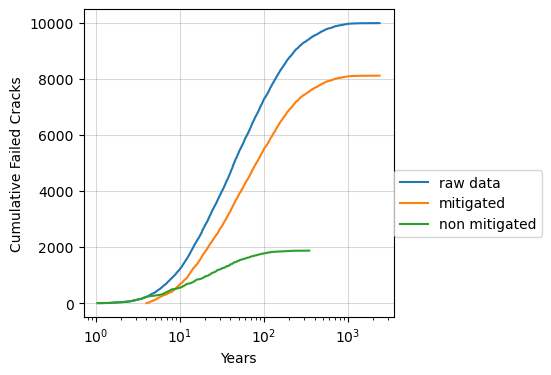